3d Molecular Designs On 2d
Abstract
Quantitative structure–activity relationship (QSAR) and quantitative structure–property relationship (QSPR) models predict biological activity and molecular property based on the numerical relationship between chemical structures and activity (property) values. Molecular representations are of importance in QSAR/QSPR analysis. Topological information of molecular structures is usually utilized (2D representations) for this purpose. However, conformational information seems important because molecules are in the three-dimensional space. As a three-dimensional molecular representation applicable to diverse compounds, similarity between a test molecule and a set of reference molecules has been previously proposed. This 3D representation was found to be effective on virtual screening for early enrichment of active compounds. In this study, we introduced the 3D representation into QSAR/QSPR modeling (regression tasks). Furthermore, we investigated relative merits of 3D representations over 2D in terms of the diversity of training data sets. For the prediction task of quantum mechanics-based properties, the 3D representations were superior to 2D. For predicting activity of small molecules against specific biological targets, no consistent trend was observed in the difference of performance using the two types of representations, irrespective of the diversity of training data sets.
Access options
Buy single article
Instant access to the full article PDF.
34,95 €
Price includes VAT (Indonesia)
Tax calculation will be finalised during checkout.
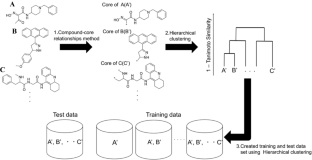

For each value of QM8 and logD of Lipophilicity, RMSE values for the test data set using SVR models and 2D, 3D descriptors are reported
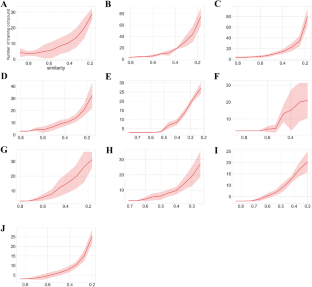
For each target, the numbers of training compounds against the diversities of training data set are reported
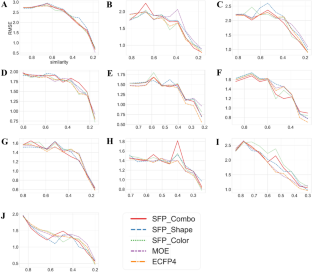
For each target macromolecule, RMSE values for the test data set against the diversity (similarity) of the training data sets are reported. Models were constructed using SVR. X-axis is the mean pairwise similarity for compounds in the training data sets

For each target macromolecule, RMSE values for the test data set using different molecular representations and kernel functions are reported. Shape_kernel + Color_kernel is represented by formula (1). Color*Shape is a hadamard product which use ColorTanimoto and ShapeTanimoto. Shape_kernel*Color_kernel is represented by formula (2)
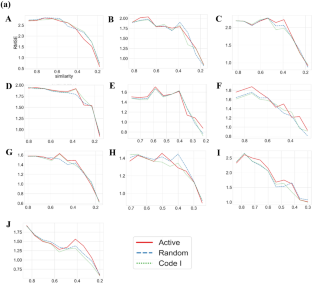
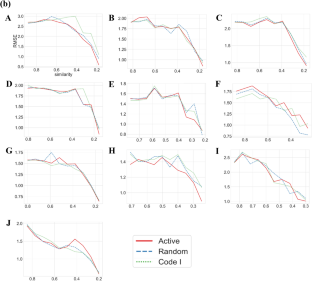
In (a), RMSE values for the test data set against training data diversity are reported. Random and Code I represent randomly extracted and Code I reference compounds besides active compounds, respectively. In (b), only individual types of compounds were used without combining active compounds
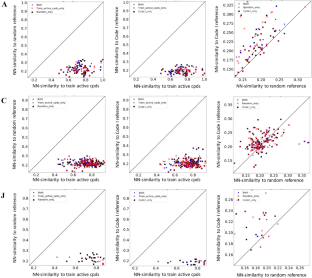
NN-distance from a test compound, which could not be predicted, to reference compounds is provided. Both represents compounds that could not be predicted by the two methods being compared. Train_active_cpds_only represents compounds that could not be predicted using only active compounds. Random_only represents compounds that could not be predicted using only randomly selected reference compounds. Code I_only represents compounds that could not be predicted using only Code I reference compounds
References
- 1.
Sippl W, Robaa D (2018) Applied chemoinformatics. Wiley-VCH Verlag GmbH & Co, KGaA, Weinheim
Google Scholar
- 2.
Rodríguez-Pérez R, Miyao T, Jasial S, Vogt M, Bajorath J (2018) Prediction of compound profiling matrices using machine learning. ACS Omega 3:4713–4723
Article Google Scholar
- 3.
Yuan Q, Wei Z, Guan X, Jiang M, Wang S, Zhang S, Li Z (2019) Toxicity prediction method based on multi-channel convolutional neural network. Molecules 24:3383
CAS Article Google Scholar
- 4.
Todeschini R, Consonni V (2009) Molecular descriptors for chemoinformatics. Methods and principles in medicinal chemistry. Wiley-VCH Verlag GmbH & Co. KGaA, Weinheim
Book Google Scholar
- 5.
Kuz'min VE, Polishchuk PG, Artemenko AG, Andronati SA (2011) Interpretation of QSAR models based on random forest methods. Mol Inf 30:593–603
Article Google Scholar
- 6.
Rodríguez-Pérez R, Bajorath J (2019) Interpretation of compound activity predictions from complex machine learning models using local approximations and shapley values. J Med Chem. https://doi.org/10.1021/acs.jmedchem.9b01101
Article PubMed Google Scholar
- 7.
Kearnes S, McCloskey K, Berndl M, Pande V, Riley P (2016) Molecular graph convolutions: moving beyond fingerprints. J Comput Aided Mol Des 30:595–608
CAS Article Google Scholar
- 8.
Jo J, Kwak B, Choi HS, Yoon S (2020) The message passing neural networks for chemical property prediction on SMILES. Methods. https://doi.org/10.1016/j.ymeth.2020.05.009
Article PubMed Google Scholar
- 9.
Cramer RD, Patterson DE, Bunce JD (1988) Comparative molecular field analysis (CoMFA). 1. Effect of shape on binding of steroids to carrier proteins. J Am Chem Soc 110:5959–5967
CAS Article Google Scholar
- 10.
Sato T, Yuki H, Takaya D, Sasaki S, Tanaka A, Honma T (2012) Application of support vector machine to three-dimensional shape-based virtual screening using comprehensive three-dimensional molecular shape overlay with known inhibitors. J Chem Inf Model 52:1015–1026
CAS Article Google Scholar
- 11.
Hu B, Kuang ZK, Feng SY, Wang D, He SB, Kong DX, Hu B, Kuang ZK, Feng SY, Wang D et al (2016) Three-dimensional biologically relevant spectrum (BRS-3D): shape similarity profile based on PDB ligands as molecular descriptors. Molecules 21:1554
Article Google Scholar
- 12.
ROCS version 3.2.2.2; OpenEye Scientific Software Inc, Santa Fe, NM.
- 13.
Hawkins PCD, Skillman AG, Nicholls A (2007) Comparison of shape-matching and docking as virtual screening tools. J Med Chem 50:74–82
CAS Article Google Scholar
- 14.
Miyao T, Jasial S, Bajorath J, Funatsu K (2019) Evaluation of different virtual screening strategies on the basis of compound sets with characteristic core distributions and dissimilarity relationships. J Comput Aided Mol Des 33:729–743
CAS Article Google Scholar
- 15.
Hu G, Kuang G, Xiao W, Li W, Liu G, Tang Y (2012) Performance evaluation of 2D fingerprint and 3D shape similarity methods in virtual screening. J Chem Inf Model 52:1103–1113
CAS Article Google Scholar
- 16.
Bento AP, Gaulton A, Hersey A, Bellis LJ, Chambers J, Davies M, Kruger FA, Light Y, Mak L, McGlinchey S, Nowotka M, Papadatos G, Santos R, Overington JP (2014) The ChEMBL bioactivity database: an update. Nucleic Acids Res 42:1083–1090
Article Google Scholar
- 17.
Naveja JJ, Vogt M, Stumpfe D, Medina-Franceo JL, Bajorath J (2019) Systematic extraction of analogue series from large compound collections using a new computational compound-core relationship method. ACS Omega 4:1027–1032
CAS Article Google Scholar
- 18.
Wu Z, Ramsundar B, Feinberg EN, Gomes J, Geniesse C, Pappu AS, Leswing K, Pande V (2018) MoleculeNet: a benchmark for molecular machine learning. Chem Sci 9:513
CAS Article Google Scholar
- 19.
Ramakrishnan R, Hartmann M, Tapavicza E, Lillienfield OAV (2015) Electronic spectra from TDDFT and machine learning in chemical space. J Chem Phys 143:084111
Article Google Scholar
- 20.
Experimental in vitro DMPK and physicochemical data on a set of publicly disclosed compounds.
- 21.
Rogers D, Hahn M (2010) Extended-connectivity fingerprints. J Chem Inf Model 50:742–754
CAS Article Google Scholar
- 22.
OEChem TK Version 2.3.0; OpeneEye Scientific Software Inc, Santa, Fe, NM
- 23.
Molecular Operating Environment (MOE) 2019.01; Chemical Computing Group ULC: 1010 Sherbooke St West Suite #910 Montreal QC Canada H3A 2R7
- 24.
OEOmega TK Version 2.9.1; OpenEye Scientific Software Inc. Santa Fe, NM
- 25.
Hornik K (1991) Approximation capabilities of multilayer feed forward networks. Neural Netw 4:251–257
Article Google Scholar
- 26.
Svetnik V, Liaw A, Tong C, Culberson JC, Sheridan RP, Feuston BP (2003) Random forest: a classification and regression tool for compound classification and QSAR modeling. J Chem Inf Comput Sci 42:1947–1958
Article Google Scholar
- 27.
Wold S, Sjostrom M, Eriksson L (2001) PLS-regression: a basic tool of chemometrics. Chemometr Intell Lab Syst 58:109–130
CAS Article Google Scholar
- 28.
Drucker H, Burges CJC, Kaufman L, Smola AJ, Vapnik V (1996) Support vector regression machines. Neural Inf Process Syst 9:155–161
Google Scholar
- 29.
Pytorch Version 1.5.0
- 30.
Optuna Version 1.3.0
- 31.
Nair V, Hinton GE (2010) Rectified linear units improve restricted Boltzmann machines. ICML 10:807–814
Google Scholar
- 32.
Chen CH, Tanaka K, Funatsu K (2018) Random forest approach to QSPR study of fluorescence properties combining quantum chemical descriptors and solvent conditions. J Fluoresc 2:695–706
Article Google Scholar
- 33.
Pedregosa F, Varoquaux G, Gramfort A, Michel V, Thirion B, Grisel O, Blondel M, Prettenhofer P, Weiss R, Dubourg V, Vanderplas J, Passos A, Cournapeau D, Brucher M, Perrot M, Duchesnay E (2011) scikit-learn: machine learning in python. J Mach Learn Res 12:2825–2830
Google Scholar
- 34.
Scipy Version 1.5.0
- 35.
Irwin JJ, Serling T, Mysinger MM, Bolstad ES, Coleman RG (2012) ZINC: a free tool to discover chemistry for biology. J Chem Inf Model 52:1757–1768
CAS Article Google Scholar
Download references
Acknowledgements
We thank OpenEye Scientific Software Inc., for providing a free academic license of the OpenEye chemistry toolkits. This work was supported by JSPS KAKENHI Grant Number JP20K19922.
Additional information
Publisher's Note
Springer Nature remains neutral with regard to jurisdictional claims in published maps and institutional affiliations.
Electronic supplementary material
Rights and permissions
About this article
Cite this article
Sato, A., Miyao, T., Jasial, S. et al. Comparing predictive ability of QSAR/QSPR models using 2D and 3D molecular representations. J Comput Aided Mol Des 35, 179–193 (2021). https://doi.org/10.1007/s10822-020-00361-7
Download citation
-
Received:
-
Accepted:
-
Published:
-
Issue Date:
-
DOI : https://doi.org/10.1007/s10822-020-00361-7
Keywords
- Quantitative structure–activity relationship
- Quantitative structure–property relationship
- Molecular representations
- Predictability of models
3d Molecular Designs On 2d
Source: https://link.springer.com/article/10.1007/s10822-020-00361-7
Posted by: gallawaysagell.blogspot.com
0 Response to "3d Molecular Designs On 2d"
Post a Comment